
As such, common deep learning user experiences that could be realized locally, would be scene detection, text recognition, object tracking and avoidance, gesturing, face recognition and natural language processing.įor instance, apps that help in organizing photos on the user's phone, utilizing an algorithm that combines artistic photography principles with deep learning technology which can sort photos based on topics, locations, and events, and can also recognize the best, based on ranking system it employees. The difference is that now all of that is going to happen locally. That includes their training too the pictures, notes, data and metadata that reside in the device will also serve to train the network and aid its learning activities such as the recognizing, ranking and classifying of objects.
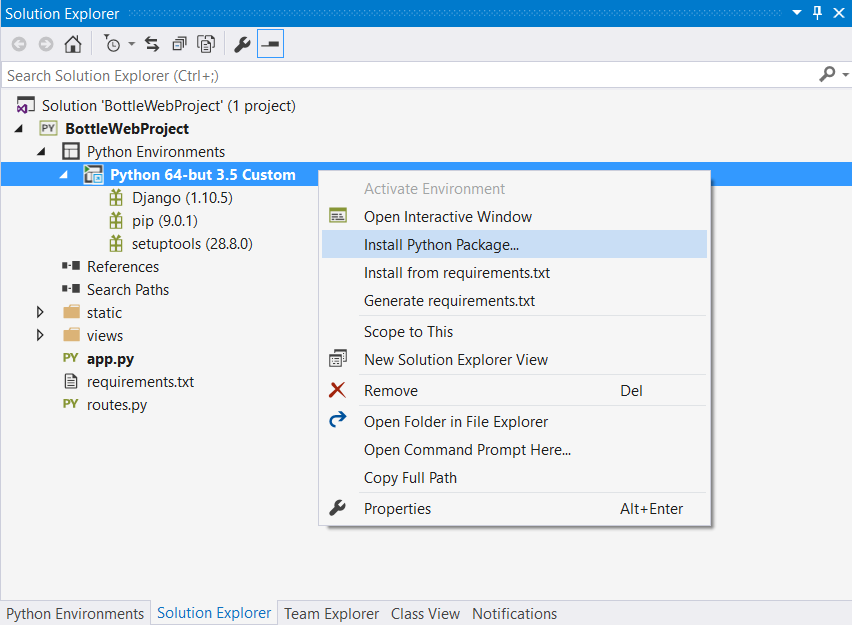
While the cloud still monopolizes the space where neural networks and their algorithms breed, things seem to be shifting with those elaborate algorithms looking to move on to and run offline on mobile devices. The extra boon is that you can use your favorite Machine Learning Python libraries like SciPy, OpenCV or TensorFlow solely on your client/mobile phone without connecting to the cloud. If you have server backend that is written in Python you can now use Python for the front end as well without paying the penalty of context switching to another tool or language, or in lacking expertise in another stack. You can pick the most suitable tool for the part of the application at hand.Įach approach has its own distinct advantages. It allows you to write Android applications in full in Python or partially, together with Java.
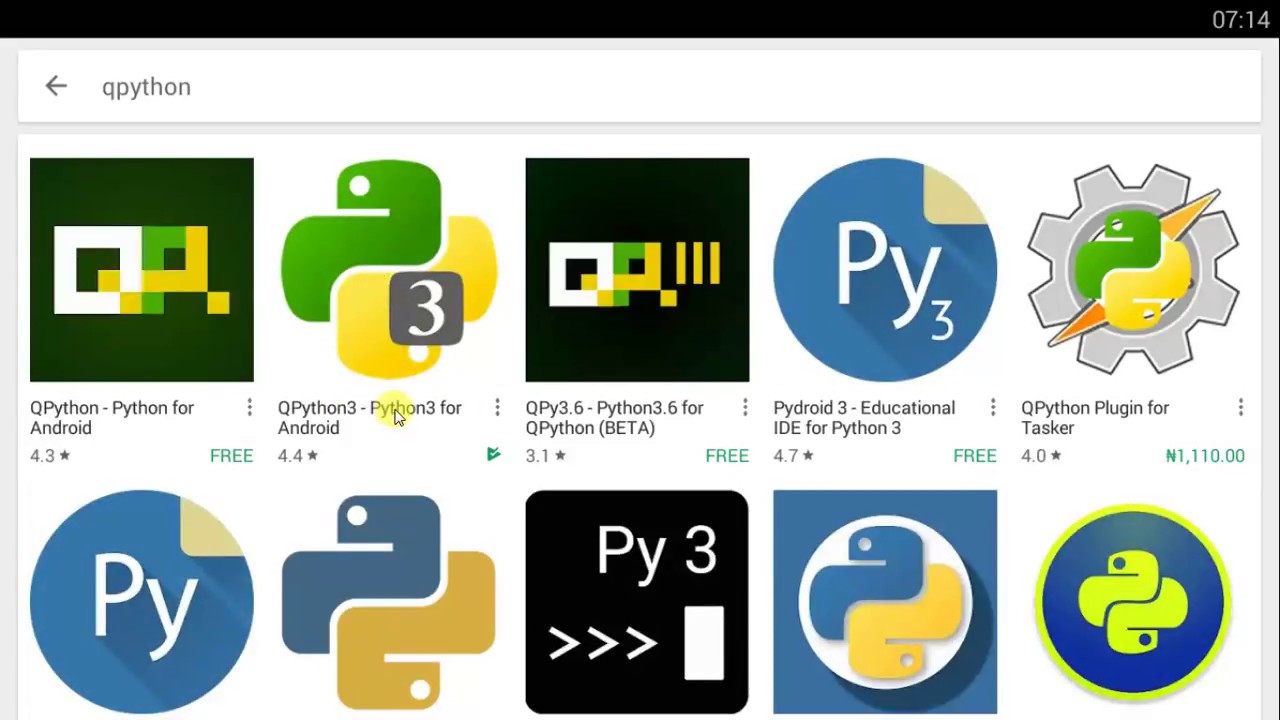
Thanks to support from Anaconda, it is now both free and open-source.Ĭhaquopy is versatile.
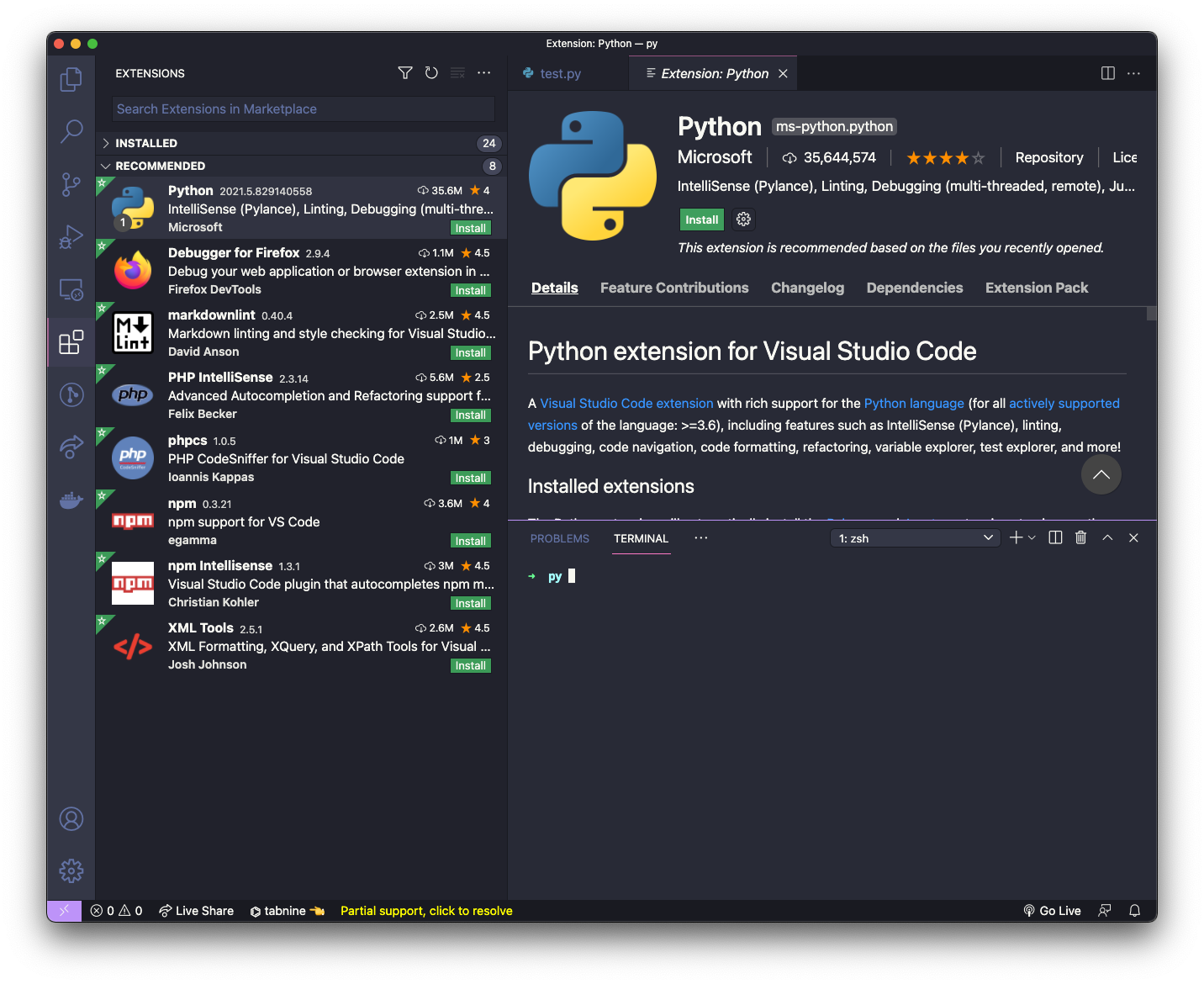
Should you choose Java or Python for your next Android project? You don't have to with Chaquopy, the Python SDK that lets you write Android applications in Python. Java Or Python For Android - Why Not Both!
